Flocking model#
Continuous-space agent interactions. Source
Each agent follows three simple rules:
maintain a minimum distance from other birds to avoid collision
fly towards the average position of neighbors
fly in the average direction of neighbors
using Agents
using Random
using LinearAlgebra
using Base64
using CairoMakie
CairoMakie.activate!(px_per_unit = 1.0)
The helper function is adapted from Agents.abmvideo
and correctly displays animations in Jupyter notebooks
function abmvio(model;
dt = 1, framerate = 30, frames = 300, title = "", showstep = true,
figure = (size = (600, 600),), axis = NamedTuple(),
recordkwargs = (compression = 23, format ="mp4"), kwargs...
)
# title and steps
abmtime_obs = Observable(abmtime(model))
if title ≠ "" && showstep
t = lift(x -> title*", time = "*string(x), abmtime_obs)
elseif showstep
t = lift(x -> "time = "*string(x), abmtime_obs)
else
t = title
end
axis = (title = t, titlealign = :left, axis...)
# First frame
fig, ax, abmobs = abmplot(model; add_controls = false, warn_deprecation = false, figure, axis, kwargs...)
resize_to_layout!(fig)
# Animation
Makie.Record(fig; framerate, recordkwargs...) do io
for j in 1:frames-1
recordframe!(io)
Agents.step!(abmobs, dt)
abmtime_obs[] = abmtime(model)
end
recordframe!(io)
end
end
abmvio (generic function with 1 method)
This agents has also three properties inherited from ContinuousAgent
id : unique identifier
pos : XY coordinate
vel: XY velocity
@agent struct Bird(ContinuousAgent{2,Float64})
speed::Float64
cohere_factor::Float64
separation::Float64
separate_factor::Float64
match_factor::Float64
visual_distance::Float64
end
Model factory function
function initialize_model(;
n_birds = 100,
speed = 1.5,
cohere_factor = 0.1,
separation = 2.0,
separate_factor = 0.25,
match_factor = 0.04,
visual_distance = 5.0,
extent = (100, 100),
seed = 2024,
)
space2d = ContinuousSpace(extent; spacing = visual_distance/1.5)
rng = Random.MersenneTwister(seed)
model = StandardABM(Bird, space2d; rng, agent_step!, scheduler = Schedulers.Randomly())
for _ in 1:n_birds
vel = rand(abmrng(model), SVector{2}) * 2 .- 1
add_agent!(
model,
vel,
speed,
cohere_factor,
separation,
separate_factor,
match_factor,
visual_distance,
)
end
return model
end
initialize_model (generic function with 1 method)
Stepping function
function agent_step!(bird, model)
# Obtain the ids of neighbors within the bird's visual distance
neighbor_ids = nearby_ids(bird, model, bird.visual_distance)
N = 0
match = separate = cohere = (0.0, 0.0)
# Calculate behaviour properties based on neighbors
for id in neighbor_ids
N += 1
neighbor = model[id].pos
heading = get_direction(bird.pos, neighbor, model)
# `cohere` computes the average position of neighboring birds
cohere = cohere .+ heading
if euclidean_distance(bird.pos, neighbor, model) < bird.separation
# `separate` repels the bird away from neighboring birds
separate = separate .- heading
end
# `match` computes the average trajectory of neighboring birds
match = match .+ model[id].vel
end
N = max(N, 1)
# Normalise results based on model input and neighbor count
cohere = cohere ./ N .* bird.cohere_factor
separate = separate ./ N .* bird.separate_factor
match = match ./ N .* bird.match_factor
# Compute velocity based on rules defined above
bird.vel = (bird.vel .+ cohere .+ separate .+ match) ./ 2
bird.vel = bird.vel ./ norm(bird.vel)
# Move bird according to new velocity and speed
move_agent!(bird, model, bird.speed)
end
agent_step! (generic function with 1 method)
Visualization#
const bird_polygon = Makie.Polygon(Point2f[(-1, -1), (2, 0), (-1, 1)])
function bird_marker(b::Bird)
φ = atan(b.vel[2], b.vel[1]) ##+ π/2 + π
rotate_polygon(bird_polygon, φ)
end
bird_marker (generic function with 1 method)
model = initialize_model()
figure, _ = abmplot(model; agent_marker = bird_marker)
figure
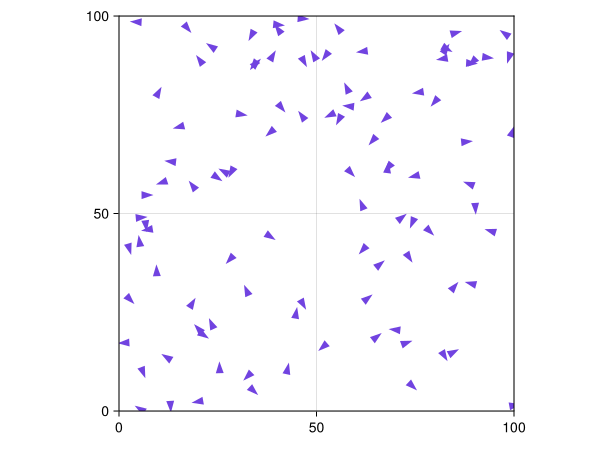
Animation
vio = abmvio(
model;
agent_marker = bird_marker,
framerate = 20, frames = 150,
title = "Flocking",
)
save("flocking.mp4", vio)
vio |> display
This notebook was generated using Literate.jl.