Solving PDEs with ModelingToolkit and NeuralPDE#
Solving Poisson PDE Systems (https://docs.sciml.ai/NeuralPDE/stable/tutorials/pdesystem/)
\[
\partial^{2}_{x}u(x,y) + \partial^{2}_{y}u(x,y) = -\sin (\pi x) \sin (\pi y)
\]
with boundary conditions
\[\begin{split}
\begin{align}
u(0, y) &= 0 \\
u(1, y) &= 0 \\
u(x, 0) &= 0 \\
u(x, 1) &= 0 \\
\end{align}
\end{split}\]
where
\(x ∈ [0, 1], y ∈ [0, 1]\)
using NeuralPDE
using Lux
using Optimization
using OptimizationOptimJL
using ModelingToolkit
using ModelingToolkit: Interval
using LineSearches
using Plots
@parameters x y
@variables u(..)
Dxx = Differential(x)^2
Dyy = Differential(y)^2
Differential(y) ∘ Differential(y)
2D PDE
eq = Dxx(u(x, y)) + Dyy(u(x, y)) ~ -sinpi(x) * sinpi(y)
\[ \begin{equation}
\frac{\mathrm{d}}{\mathrm{d}y} \frac{\mathrm{d}}{\mathrm{d}y} u\left( x, y \right) + \frac{\mathrm{d}}{\mathrm{d}x} \frac{\mathrm{d}}{\mathrm{d}x} u\left( x, y \right) = - sinpi\left( x \right) sinpi\left( y \right)
\end{equation}
\]
Boundary conditions
bcs = [
u(0, y) ~ 0.0,
u(1, y) ~ 0.0,
u(x, 0) ~ 0.0,
u(x, 1) ~ 0.0
]
\[\begin{split} \begin{align}
u\left( 0, y \right) &= 0 \\
u\left( 1, y \right) &= 0 \\
u\left( x, 0 \right) &= 0 \\
u\left( x, 1 \right) &= 0
\end{align}
\end{split}\]
Space domains
domains = [
x ∈ Interval(0.0, 1.0),
y ∈ Interval(0.0, 1.0)
]
2-element Vector{Symbolics.VarDomainPairing}:
Symbolics.VarDomainPairing(x, 0.0 .. 1.0)
Symbolics.VarDomainPairing(y, 0.0 .. 1.0)
Build a neural network for the PDE solver.
Input: 2 dimensions.
Hidden layers: 16 neurons * 2 layers.
Output: single output u(x, y)
dim = 2
chain = Lux.Chain(Dense(dim, 16, Lux.σ), Dense(16, 16, Lux.σ), Dense(16, 1))
Chain(
layer_1 = Dense(2 => 16, σ), # 48 parameters
layer_2 = Dense(16 => 16, σ), # 272 parameters
layer_3 = Dense(16 => 1), # 17 parameters
) # Total: 337 parameters,
# plus 0 states.
Discretization method usesPhysicsInformedNN()
(PINN).
dx = 0.05
discretization = PhysicsInformedNN(chain, QuadratureTraining(; batch = 200, abstol = 1e-6, reltol = 1e-6))
NeuralPDE.PhysicsInformedNN{Lux.Chain{@NamedTuple{layer_1::Lux.Dense{typeof(NNlib.σ), Int64, Int64, Nothing, Nothing, Static.True}, layer_2::Lux.Dense{typeof(NNlib.σ), Int64, Int64, Nothing, Nothing, Static.True}, layer_3::Lux.Dense{typeof(identity), Int64, Int64, Nothing, Nothing, Static.True}}, Nothing}, NeuralPDE.QuadratureTraining{Float64, Integrals.CubatureJLh}, Nothing, NeuralPDE.Phi{Lux.StatefulLuxLayer{Static.True, Lux.Chain{@NamedTuple{layer_1::Lux.Dense{typeof(NNlib.σ), Int64, Int64, Nothing, Nothing, Static.True}, layer_2::Lux.Dense{typeof(NNlib.σ), Int64, Int64, Nothing, Nothing, Static.True}, layer_3::Lux.Dense{typeof(identity), Int64, Int64, Nothing, Nothing, Static.True}}, Nothing}, Nothing, @NamedTuple{layer_1::@NamedTuple{}, layer_2::@NamedTuple{}, layer_3::@NamedTuple{}}}}, typeof(NeuralPDE.numeric_derivative), Bool, Nothing, Nothing, Nothing, Base.RefValue{Int64}, Base.Pairs{Symbol, Union{}, Tuple{}, @NamedTuple{}}}(Lux.Chain{@NamedTuple{layer_1::Lux.Dense{typeof(NNlib.σ), Int64, Int64, Nothing, Nothing, Static.True}, layer_2::Lux.Dense{typeof(NNlib.σ), Int64, Int64, Nothing, Nothing, Static.True}, layer_3::Lux.Dense{typeof(identity), Int64, Int64, Nothing, Nothing, Static.True}}, Nothing}((layer_1 = Dense(2 => 16, σ), layer_2 = Dense(16 => 16, σ), layer_3 = Dense(16 => 1)), nothing), NeuralPDE.QuadratureTraining{Float64, Integrals.CubatureJLh}(Integrals.CubatureJLh(0), 1.0e-6, 1.0e-6, 1000, 200), nothing, NeuralPDE.Phi{Lux.StatefulLuxLayer{Static.True, Lux.Chain{@NamedTuple{layer_1::Lux.Dense{typeof(NNlib.σ), Int64, Int64, Nothing, Nothing, Static.True}, layer_2::Lux.Dense{typeof(NNlib.σ), Int64, Int64, Nothing, Nothing, Static.True}, layer_3::Lux.Dense{typeof(identity), Int64, Int64, Nothing, Nothing, Static.True}}, Nothing}, Nothing, @NamedTuple{layer_1::@NamedTuple{}, layer_2::@NamedTuple{}, layer_3::@NamedTuple{}}}}(Lux.StatefulLuxLayer{Static.True, Lux.Chain{@NamedTuple{layer_1::Lux.Dense{typeof(NNlib.σ), Int64, Int64, Nothing, Nothing, Static.True}, layer_2::Lux.Dense{typeof(NNlib.σ), Int64, Int64, Nothing, Nothing, Static.True}, layer_3::Lux.Dense{typeof(identity), Int64, Int64, Nothing, Nothing, Static.True}}, Nothing}, Nothing, @NamedTuple{layer_1::@NamedTuple{}, layer_2::@NamedTuple{}, layer_3::@NamedTuple{}}}(Lux.Chain{@NamedTuple{layer_1::Lux.Dense{typeof(NNlib.σ), Int64, Int64, Nothing, Nothing, Static.True}, layer_2::Lux.Dense{typeof(NNlib.σ), Int64, Int64, Nothing, Nothing, Static.True}, layer_3::Lux.Dense{typeof(identity), Int64, Int64, Nothing, Nothing, Static.True}}, Nothing}((layer_1 = Dense(2 => 16, σ), layer_2 = Dense(16 => 16, σ), layer_3 = Dense(16 => 1)), nothing), nothing, (layer_1 = NamedTuple(), layer_2 = NamedTuple(), layer_3 = NamedTuple()), nothing, static(true))), NeuralPDE.numeric_derivative, false, nothing, nothing, nothing, NeuralPDE.LogOptions(50), Base.RefValue{Int64}(1), true, false, Base.Pairs{Symbol, Union{}, Tuple{}, @NamedTuple{}}())
Build the PDE system and discretize it.
@named pde_system = PDESystem(eq, bcs, domains, [x, y], [u(x, y)])
prob = discretize(pde_system, discretization)
OptimizationProblem. In-place: true
u0: ComponentVector{Float64}(layer_1 = (weight = [0.8196878433227539 0.7004758715629578; 0.3931356370449066 0.48712244629859924; … ; 0.449299156665802 0.8960288166999817; 0.9092181324958801 -0.6197015643119812], bias = [-0.15974102914333344, 0.6883767247200012, -0.48731139302253723, 0.1613699197769165, -0.6321574449539185, 0.3698040246963501, -0.5370475053787231, 0.22827871143817902, 0.5548525452613831, 0.3299241065979004, 0.5423669815063477, 0.1224275752902031, -0.3971497416496277, 0.11908895522356033, -0.017739862203598022, -0.30539336800575256]), layer_2 = (weight = [0.22286070883274078 -0.1559523493051529 … 0.27038872241973877 -0.11212626099586487; 0.02108357846736908 -0.39513230323791504 … -0.09282369911670685 0.2834043800830841; … ; -0.016988269984722137 0.0362246073782444 … 0.20843735337257385 -0.10103217512369156; -0.04013289883732796 -0.21068252623081207 … -0.07309538125991821 0.25288477540016174], bias = [-0.1563253402709961, -0.1046404242515564, -0.08406564593315125, 0.00858280062675476, 0.03033331036567688, -0.10524767637252808, 0.14995041489601135, -0.2301800549030304, 0.1252993941307068, 0.09505137801170349, -0.18562549352645874, -0.19945639371871948, 0.054662466049194336, 0.024142414331436157, 0.19192862510681152, -0.002922743558883667]), layer_3 = (weight = [0.3946394920349121 0.20195671916007996 … -0.20656079053878784 0.2948485016822815], bias = [0.15253832936286926]))
Callback function to record the loss
lossrecord = Float64[]
callback = function (p, l)
push!(lossrecord, l)
return false
end
#1 (generic function with 1 method)
Solve the problem. It may take a long time.
opt = OptimizationOptimJL.LBFGS(linesearch = LineSearches.BackTracking())
res = Optimization.solve(prob, opt, callback = callback, maxiters=1000)
retcode: Success
u: ComponentVector{Float64}(layer_1 = (weight = [1.5071675070120205 0.8823034566579928; 0.5636273086279047 0.6832895430415558; … ; 0.5979174592213216 0.8991062665856987; 1.0557566343891165 -0.6861942078676764], bias = [-0.1196047545469051, 0.24607137396682394, -0.9819751544126905, 0.8624041980707824, -1.0169017291740878, -0.004594491175763093, -3.0945086684301537, -0.696834011732565, 0.6900372231392305, -0.42455507183566554, 0.08384554759511069, 0.738188530137531, -0.7745059513448691, 0.5259545266473501, 0.042915033024530966, -1.6413278648413172]), layer_2 = (weight = [0.1706422652260948 -0.053810692090416626 … 0.2328455101197059 0.3662537747158219; 0.08360503070093009 -0.38699552453871305 … -0.025527547430370494 0.2470275574975936; … ; -0.2234395055634697 0.08748509593227435 … 0.13689783857151344 -0.09506753891284882; -0.05448726440326345 0.04424084662996196 … -0.02968893958911444 0.7963135028375397], bias = [0.3985794445142187, -0.079272923559492, 0.5414622921266845, 0.0943138825167683, -0.020904091241865673, -0.10768171483985353, -0.012427236243366854, -0.3143427447533921, 0.016356222292157267, 0.24502652896393654, -0.28078923205563305, -0.27213817091525433, 0.07906242934582597, -0.10541984174778792, 0.07480765601995526, -0.006963238914553712]), layer_3 = (weight = [1.7679566771166069 0.0801626953576281 … 0.9661879971188425 0.9713577066548346], bias = [0.3025539447848016]))
plot(lossrecord, xlabel="Iters", yscale=:log10, ylabel="Loss", lab=false)
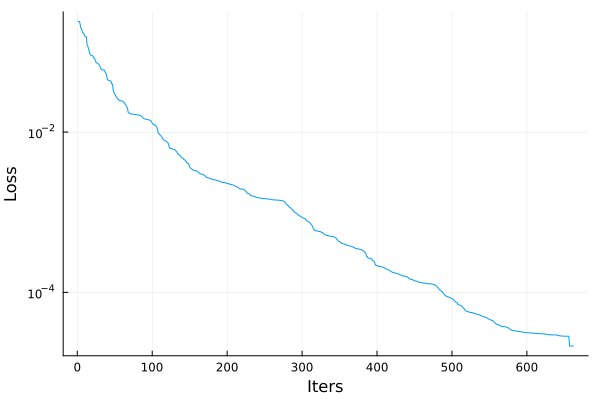
Plot the predicted solution of the PDE and compare it with the analytical solution to see the relative error.
xs, ys = [infimum(d.domain):dx/10:supremum(d.domain) for d in domains]
analytic_sol_func(x,y) = (sinpi(x)*sinpi(y))/(2pi^2)
phi = discretization.phi
u_predict = reshape([first(phi([x, y], res.u)) for x in xs for y in ys], (length(xs), length(ys)))
u_real = reshape([analytic_sol_func(x, y) for x in xs for y in ys], (length(xs), length(ys)))
diff_u = abs.(u_predict .- u_real)
201×201 Matrix{Float64}:
0.00353296 0.00349458 0.00345502 0.00341432 … 0.00156265 0.00162071
0.00337825 0.00334323 0.00330702 0.00326963 0.00153785 0.00159432
0.00322543 0.00319372 0.00316078 0.00312664 0.00151277 0.00156769
0.00307451 0.00304605 0.00301632 0.00298536 0.00148745 0.00154085
0.00292549 0.00290021 0.00287364 0.0028458 0.00146191 0.00151382
0.00277837 0.00275621 0.00273273 0.00270795 … 0.00143618 0.00148664
0.00263317 0.00261406 0.00259361 0.00257183 0.00141028 0.00145932
0.00248987 0.00247376 0.00245627 0.00243744 0.00138424 0.00143189
0.00234848 0.00233531 0.00232073 0.00230477 0.0013581 0.00140438
0.00220901 0.00219871 0.00218698 0.00217385 0.00133186 0.00137681
⋮ ⋱ ⋮
0.00222347 0.00215996 0.00209766 0.00203657 0.00095197 0.000979465
0.00226464 0.00219936 0.00213533 0.00207257 0.000963349 0.000991422
0.00230508 0.00223801 0.00217226 0.00210781 0.000974381 0.00100303
0.0023448 0.00227593 0.00220843 0.00214228 … 0.000985048 0.00101426
0.00238379 0.00231311 0.00224385 0.00217599 0.000995332 0.00102511
0.00242204 0.00234954 0.0022785 0.00220893 0.00100521 0.00103555
0.00245956 0.00238522 0.0023124 0.0022411 0.00101468 0.00104556
0.00249635 0.00242016 0.00234554 0.00227249 0.0010237 0.00105513
0.00253241 0.00245434 0.00237792 0.00230312 … 0.00103227 0.00106423
p1 = plot(xs, ys, u_real, linetype=:contourf, title = "analytic");
p2 = plot(xs, ys, u_predict, linetype=:contourf, title = "predicted");
p3 = plot(xs, ys, diff_u, linetype=:contourf, title = "error");
plot(p1, p2, p3)
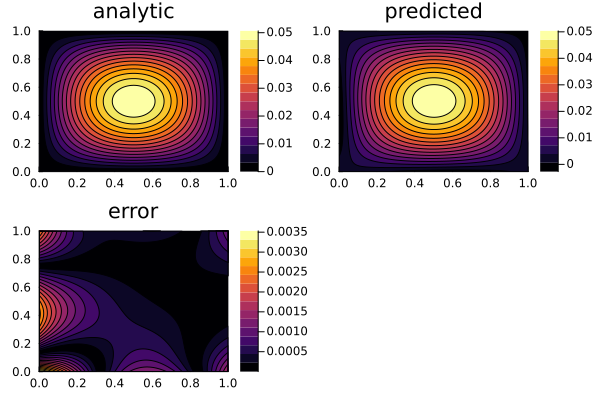
This notebook was generated using Literate.jl.